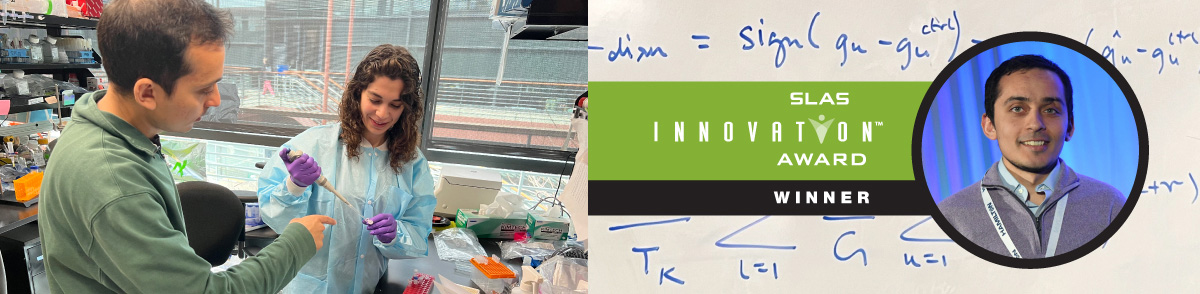
May 8, 2023
Understanding how cells respond to genetic perturbation can have an enormous impact on a wide array of biomedical applications – from identifying the causes of cancer drug resistance to improved precision in re-engineering cells. Yusuf Roohani, M.Sc., leverages the powers of deep learning and biological knowledge graphs to build computational models for in-silico gene perturbation that help scientists maximize the value generated by large perturbational screens.
“Even the name of our computational method, GEARS, captures the imagery of the cell’s machinery,” says Roohani, a Stanford University (Stanford, CA, USA) Ph.D. student advised by Jure Leskovec, Ph.D., and Stephen Quake, Ph.D. The Graph-Enhanced Gene Activation and Repression Simulator (GEARS) is a deep-learning method that uses single-cell RNA sequencing data from perturbational experiments along with a network of gene-gene relationships to predict the transcriptional response to genetic perturbation.
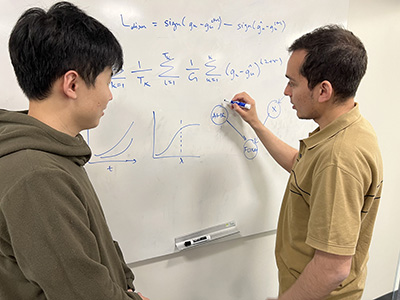
Large-scale CRISPR-based perturbational screens are an important tool for uncovering insights into cellular function. Recent developments have increased both the precision with which genes can be targeted as well as the scale of information generated from each experiment (e.g., the Perturb-Seq assay, which measures the full transcriptional state following perturbation). However, there is still a problem of deciding which genes to perturb. Perturbing all possible combinations of genes in a high-content phenotypic screen is slow, laborious and expensive. While computational modeling can help navigate the vast space of combinatorial perturbations and prioritize experiments, existing approaches face many limitations in fulfilling this potential. Most notably, they are unable to effectively predict outcomes of perturbing novel genes not experimentally perturbed, and they struggle at detecting interactions between genes following combinatorial perturbation.
Enter the GEARS model. GEARS uses what is already known about genes and their function to predict the transcriptional outcome of perturbing genes that may never have been experimentally perturbed before. By representing each gene with its own embedding (a unique list of numbers used for computational operations), GEARS is also able to predict emergent interactions between genes and capture the heterogeneity in their perturbation response. This is similar to how large language models (like ChatGPT) use word embeddings to understand language better.
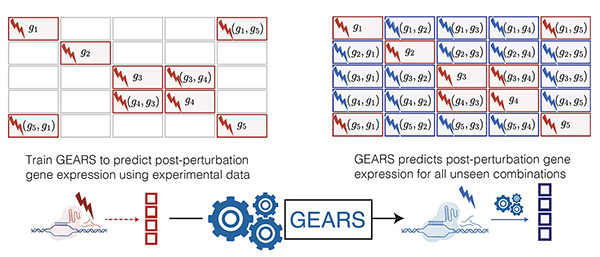
“The GEARS computational model simulates the gene expression response of a cell when certain genes have been knocked out or activated,” explains Roohani. “So, when you do some kind of gene editing, such as CRISPRa or CRISPRi, GEARS can help predict how the cell will respond in terms of gene expression. GEARS is meant to be a tool that can help a wide array of biomedical scientists from those working in drug discovery to cancer biology to regenerative medicine.”
Roohani, along with co-authors Leskovec and Kexin Huang, use GEARS to perform in-silico genetic perturbations and prioritize future experiments to maximize the information obtained at minimum experimental cost. Roohani expects tools such as GEARS to be invaluable for accelerating the discovery of more effective and patient-specific therapeutics.
“CRISPR-based screening is very popular both in pharma and some academic labs as a scalable approach to interrogate various different phenotypes that might be relevant to a specific disease,” says Roohani, who believes that GEARS is uniquely positioned to significantly expand the information gained from these phenotypic screens. “GEARS can computationally uncover a much larger set of perturbation outcomes than was previously possible using the same experimental data.”
The novel method earned Roohani the SLAS Innovation Award at the SLAS2023 International Conference and Exhibition, where he delved into its potential in his award-winning presentation, “GEARS: Predicting Transcriptional Outcomes of Novel Multi-gene Perturbations.”
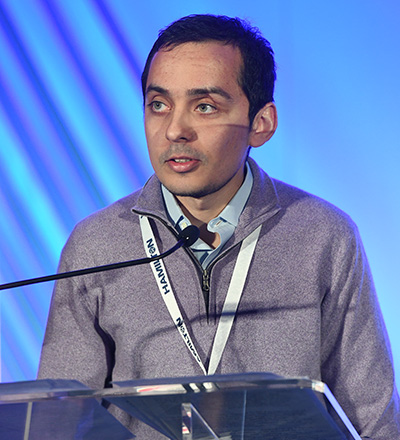
Before starting his Ph.D. program, Roohani became familiar with the challenges and opportunities surrounding large data sets as a machine learning engineer at GSK working with high-throughput (HTS) and high content screening (HCS). “The experience helped me understand questions scientists are trying to answer and challenges they face in getting the most out of all the data that they're generating,” says Roohani, who led a cross-disciplinary team to biologically profile GSK’s two-million-plus compound collection using multi-modal datasets and HTS.
“Data is very abundant within the drug discovery world. It's definitely not a constraint. What ends up being the constraint is drawing meaningful insight from the data,” he continues. “As you look at the data, you realize that there's a lot of redundancy in how cells react to different interventions because cells have underlying mechanisms by which they respond. Those are quite well defined for large sets of interventions. You can leverage these intuitions to make models that identify patterns and make inferences.”
Roohani, who resets his mindset by getting into nature and doing a bit of running or biking and prioritizes time spent at the park with his two-year-old son, Musa, is excited about the visibility and potential for collaboration that the SLAS Innovation Award is giving to his research. He also is grateful for the exposure to the entire drug discovery process that he gained while working in the pharmaceutical industry.
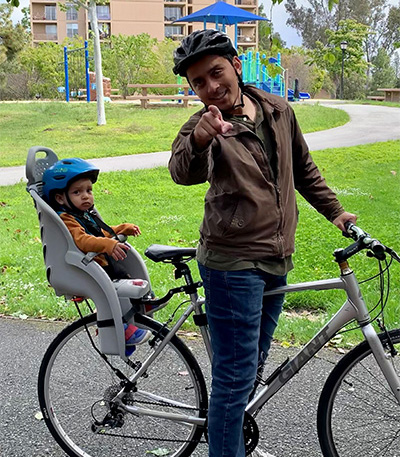
“Many people in academia aren't even aware of challenges faced within the screening world, because very few academic labs have access to that kind of infrastructure,” he says. “They're often not aware of the sort of scaling and other cost challenges that you have with it. I was definitely motivated by my time in industry. Research is not just identifying a problem; it’s also crafting solutions into a useful, translational form.”
He combined his curiosity and interests into a Ph.D. program examining AI applied to biomedical data within the drug discovery space, a move that eventually inspired the development of GEARS. From the start, Roohani was interested in leveraging the power of computation for advancing scientific discovery.
“I have always been interested in how to use computational models of the physical world to predict new phenomena or to simply understand how the world operates. In the context of cell biology, these models can search for optimal therapeutic interventions at a scale that is impossible with physical experimentation,” he says.
Ongoing collaborations with other academic labs at Stanford are exploring the use of GEARS across diverse biological contexts. “We're excited to see the outcomes of those lab experiments and learning more as we share this technology with more groups and researchers – for instance, how can GEARS be made more generalizable across cell types or how do we increase robustness to noisy single-cell data sets,” says Roohani, who adds that he is personally interested in the problem of re-engineering cells and cell-therapy based applications. “I knew you could gain insights from perturbation response about the functioning of the cell. What I was curious about was how AI could help identify the best perturbations to reconfigure cells.
“I think scientists involved in running HTS and functional genomics screens would be interested in GEARS. Computational scientists should have no trouble getting started with the open-source version of our tool that's available online,” says Roohani.
Sidelines
Unveiling the Capabilities of AI in Transforming Drug Development – Read About It in SLAS Technology