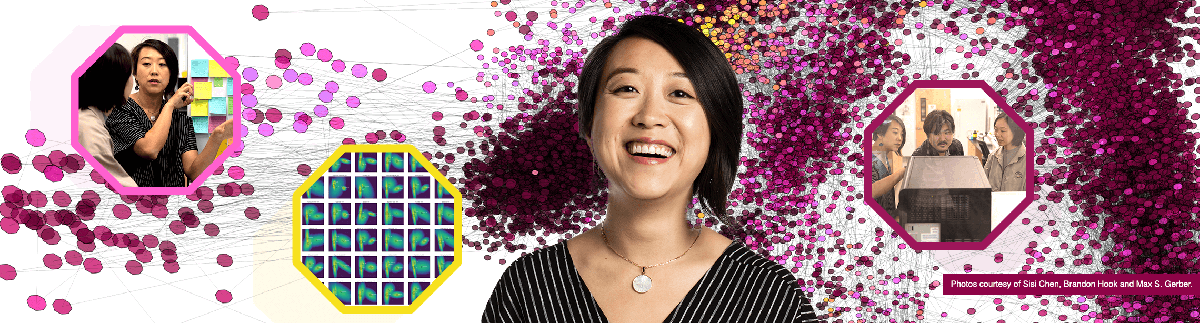
April 9, 2021
Siyu (Sisi) Chen, Ph.D., developed a computational framework for analyzing data across hundreds of samples to better understand heterogeneous cell populations in a wide range of therapeutic and disease conditions. To describe how her team pursues disease progression, she borrows a hockey analogy from Wayne Gretzky: Don’t go where the puck is now, go where it will be next.
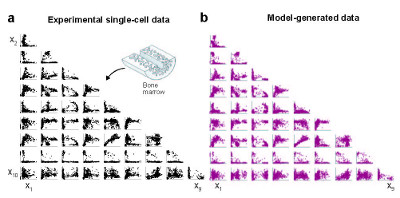
“We use the whole platform to examine how drugs steer the immune system and how cell populations change in response to drugs, signals and genetics,” Chen explains. “As we developed our platform, we realized that we had an opportunity to move to the next level. We wanted to engineer strategies that push the entire cell population to states useful for disease resolution and to create better regenerative therapies.”
Her intuitive modeling framework gathers single-cell data using highly multiplexed single-cell mRNA-seq to study the impact of over 500 immunomodulatory compounds on human primary blood mononuclear cells (PBMCs), a heterogenous mixture of myeloid and lymphoid immune cell-types. To date, her platform has profiled over one million single cells by using MULTI-seq to multiplex samples and a probabilistic modeling platform called PopAlign to discover cell-type specific responses for each compound in the library. PopAlign scales to comparisons involving tens to hundreds of samples, enabling large-scale studies of changes in natural and engineered cell populations.
Chen’s team plans a number of applications for the platform, which is poised to become a valuable resource for improving therapeutic strategies, especially in cancer where the macrophage state (M1/M2) can advance or reverse disease progression, including modeling and predicting how drug combinations impact the immune system, drug efficacies, identifying function for natural products and predicting new molecular structures that might impact the system.
“We know that chemical space is infinitely large and the richness of this data can help us develop models to predict new chemistries that might be able to produce biological impacts within a complex system,” she comments, adding that she also is interested in mining genetic or signaling perturbations from the data that can help produce better therapeutic cells for brain tissue repairs.
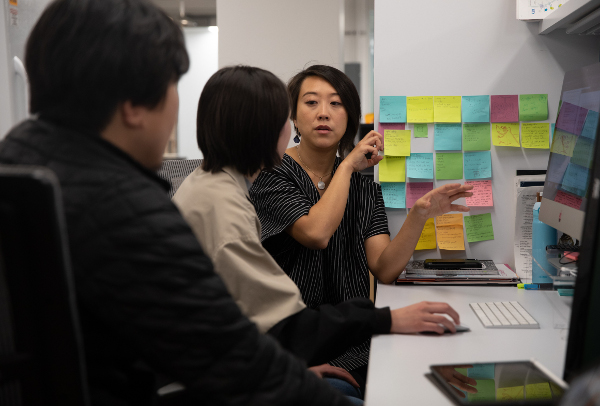
“I think that previous frameworks for predicting combination effects are usually on one output so you measure one function, such as growth rate or a particular gene, and you try to predict how combinations will work. Now we have the opportunity to build a new framework based on all of this complex data to predict how combinations will work together,” says Chen.
“I think one of the striking things is that all of these different drugs only have six different classes of behavior. Even though you’re hitting different target proteins within the immune system, you’re limited in terms of the effect that you can have on the transcriptional state,” Chen says. “This leads to the question, if you use combinations, can you produce more distinct modes of behavior?”
Chen describes the basic idea as modeling the entire population of cells as a single mathematical entity, capturing a highly dimensional state of different subpopulations and encapsulating those areas as a local probability density using Gaussian mixture models. Chen’s team dissects the data hierarchically, first exploring changes from drugs and perturbations across the entire population, then examining subpopulation differences and then taking it another step down to see how specific genes react.
“The fact that we have this whole integrated framework for every sample is unique,” says Chen, director of the Single Cell Profiling and Engineering Center (SPEC) at the California Institute of Technology (Caltech, Pasadena, CA, USA). “Most analysis techniques are focused on putting everything together and then you have collections of subpopulations that are not built for comparing across samples. Our framework is built for comparing what changes across the entire sample.”
The breakthrough innovation represents collaborative efforts between Chen’s team at Caltech and a team at the University of California, San Francisco (UCSF, USA). The framework holds the potential to expand research when paired with other technology and earned Chen the 2021 SLAS Innovation Award at the SLAS2021 Digital International Conference and Exhibition. She unpacked the promising dataset in her award-winning presentation, “Defining the Breadth and Specificity of Drug Response in Heterogeneous Immune Cell Populations.”
A Fascination with Single-Cell Function
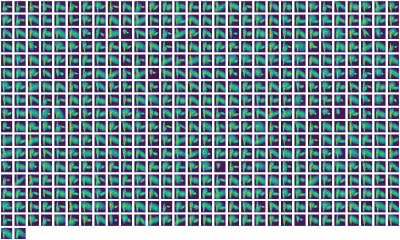
What captivates Chen about single cell biology is how individual cells decide what fate to take on and how they choose which function to pursue in the face of conflicting instructions. Chen explored this question throughout her graduate school years at University of California, Berkeley, (UC Berkeley, USA), where she earned her Ph.D. in bioengineering.
“I felt like I was in a data desert at that time,” Chen comments. “To complete every graph, you had to spend an entire workday counting immuno-stained cells one by one.” She decided there had to be a better way.
“This work wasn’t quantifiable, it wasn’t reproducible and that’s what led me to single cell transcriptional profiling, and the work I showed at SLAS. I realized that we need extremely quantitative measurements that tell us what the cell is doing on a molecular level in order to understand how cells differentiate and what functions they take on,” says Chen. In 2015, Chen took at post-doc opportunity in the lab of Matt Thomson, Ph.D., at the UCSF Department of Pharmacology to study how to apply the right perturbations to stem cells to push them into defined states. “Single cell measurements were a great way of doing that,” Chen says, adding that the team decided early on to build a whole system from the ground up.
“What was so interesting is that we weren’t even sure whether we could get data using the methods we pursued. For example, I was pipetting single cells into microchambers to see if I could extract signal – very hokey, scrappy stuff,” she reports, adding: “Research is messy. Many factors come into play and also technologies rapidly change. You’re always adapting in response to what’s possible and what’s being done.”
Influenced by published papers on single cell profiling, including one technique called Drop-seq, Chen and Thomson collaborated on a droplet-based system developed by the microfluidic group of Adam Abate, Ph.D., at UCSF, and focused on a computational framework to solve a multitude of microfluidic problems from synthesis errors on the beads, to computational ways to account for these errors, to data analysis, Chen explains. The first hurdle was cultivating enough samples.
“I developed this computational framework for analyzing data across hundreds of samples, but at the time, there weren’t hundreds of samples,” Chen explains. “Now, it’s possible to profile as many samples as we need, and everyone’s going to look for opportunities to expand research with the new multiplexing technologies that have come out over the last two years, including one that I personally had a hand in developing.” She refers to her work with Caltech colleagues Lior Pachter, Ph.D., and Jase Gehring, Ph.D., on ClickTags, a universal sample multiplexing method for single-cell RNA sequencing in which fixed cells are chemically labeled by attaching identifying DNA oligonucleotides to cellular proteins.
Finding Partners to Expand Potential
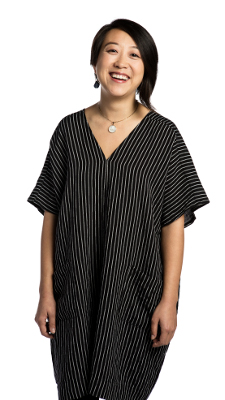
Chen emphasizes the need to collaborate with researchers in other areas of expertise when developing new computational tools. “Technology stacks today are so complex. You need people at every layer to collect and interpret data. You need people who understand the biology, the experiment and its molecular details, the sequencing and its limitations, and then how to compute over the data and chemistry,” she continues. “There’s a huge array of knowledge and technology that goes into executing one set of experiments. I don’t think it’s possible to do research without other developers. Had I taken that approach, I would have been building something on my own without any application.”
She is excited about connecting with people from other groups represented within the SLAS community. “I know there are those who believe that you need to have total freedom from commercial and practical demands to do effective research,” Chen observes. “While that may be true for some, I think a lot of people want to see their work translated. What’s so beautiful about the SLAS conference is that it shows you how people are doing that. This is the first year I have been involved with SLAS, but it won’t be my last.”
Chen received many emails from researchers eager to engage with her since receiving top honors in January. She also has been in touch with 2020 SLAS Innovation Award winner Joe de Rutte, Ph.D. “I talked to Joe about his lab on a particle approach that would be interesting to combine with our platform. With those types of systems you could do an even higher order of perturbation, so that’s something that I’m interested in connecting with our technology,” says Chen.
“Computation is a huge part of technology development and it’s imperative that people continue to build new computational tools for analyzing this incredible wealth of data now available,” she continues. “The PopAlign framework so far is an amazing first step, and we’re going to continue building new computational tools for doing predictions. It’s insane how quickly we’ve moved from the data desert to being underwater with the flood of information!”
Seeking Science Perspectives
In spite of her early interest in biology – she attended many biology summer camps in her youth and even volunteered at a snake venom research lab in high school – Chen chose to study electrical engineering during her undergraduate years at Massachusetts Institute of Technology (MIT, Cambridge, MA, USA).
“I wanted a quantitative background to learn more math and have this set of universal skills. However, what I discovered as an undergraduate was that electrical systems didn’t inspire me much,” she laughs. “The principles are great, but I couldn’t foresee myself designing silicon circuits. So I returned to biology, which felt like the next great scientific frontier.”
However, shifting gears from engineering to biology was hard, she says. “In biology everything you learn is very context dependent,” Chen explains. “In engineering, the principles you learn tend to be more stackable. You learn one concept and map that on top of another concept. In biology, a lot of the concepts – such as which protein has what effect – is context dependent. It was much more difficult to link things together, especially before we had these single-cell measurements that could provide a systems-level overview.”
To make a smoother transition to bioengineering, Chen realized and her graduate committee recommended, that she needed to learn more biology. Therefore she enrolled in the famous marine biology physiology course at Woods Hole, MA, USA, and later pursued studies at Caltech with Rob Phillips, Ph.D., and Wallace Marshall, Ph.D., who, among others, continue to mentor Chen today. “These pivotal opportunities made me fall in love with biology again,” she comments. “Being surrounded by so many talented people was motivational.”
During these years Chen also cultivated an interest in the arts, expanding her study of single cells beyond the lab and into the pottery studio. While her early ceramic creations ranged from thrown vessels to a bust on display at the Caltech Diversity and Art Fall 2020 collection, she is currently making ceramic sculptures inspired by cells.
“I call it the smallest-scale, lowest throughput ‘single-cell atlas’ because I make one cell every two months!” she laughs. “I don’t seem to ever have time to get to the studio between my work schedule and the shelter-in-place observations of the past year. Ceramics are a cool way for me to think about cellular architecture. I’m hoping that it will give me another view into what cells are doing.
“Ceramics are so tactile. It keeps me sane to have something that is concrete when work is not,” she observes. “I’ve learned a lot about shaping, planning and experimenting through my ceramic projects. There are stages you go through, so it’s a lot like science from a different perspective.”
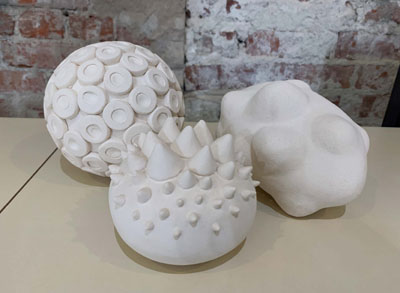
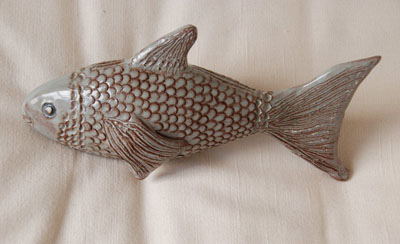
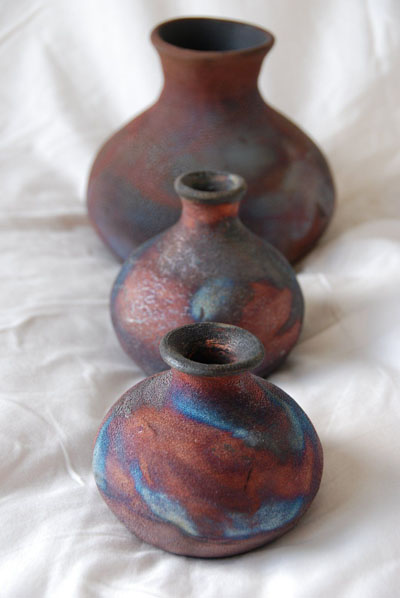
Sidelines
Hear Chen on SLAS New Matter: Inside the Minds of SLAS Scientists
Learn More about the SLAS Innovation Award and 2021 Finalists!